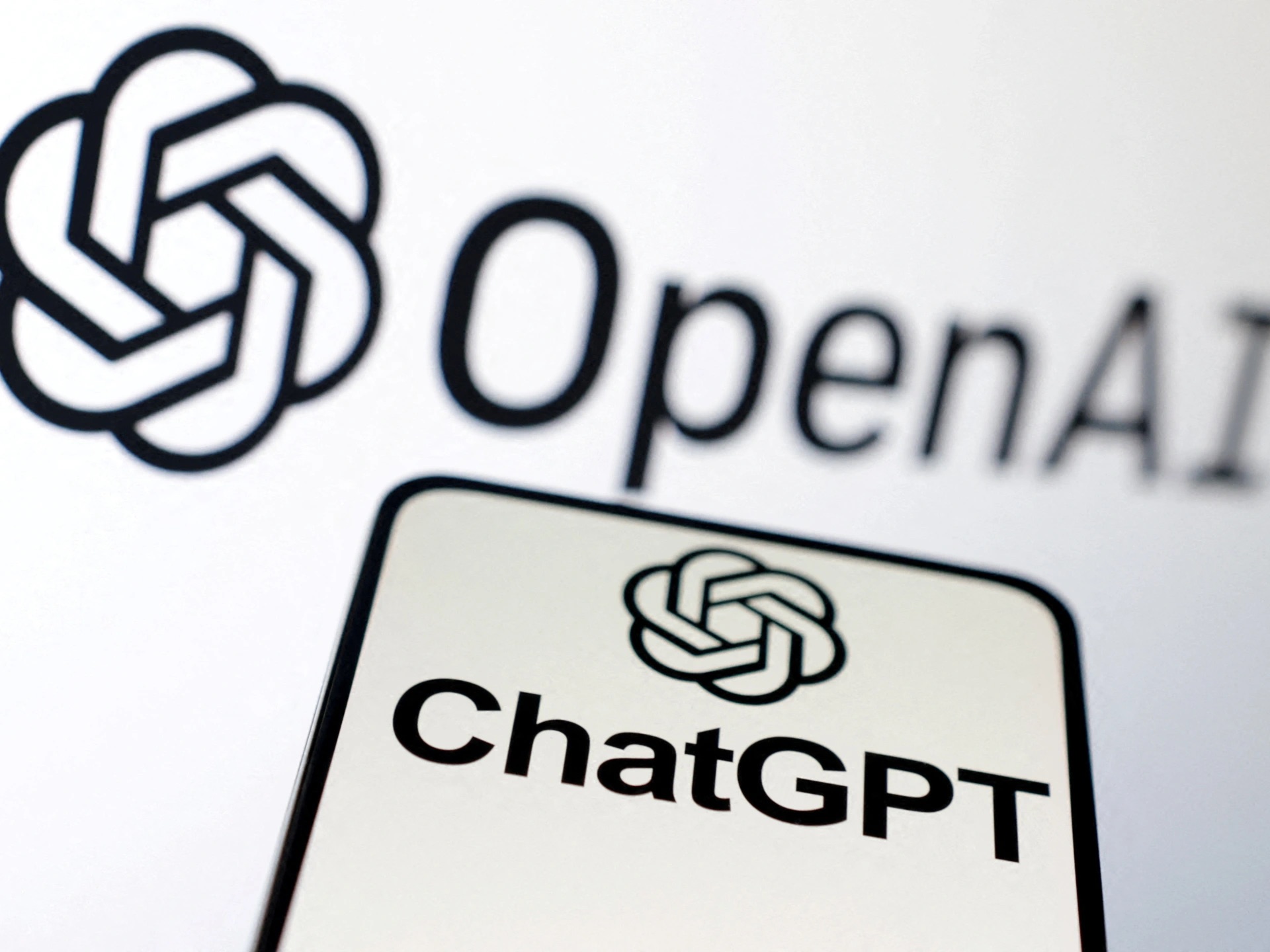
Artificial Intelligence in finance today
13 min read
So, this is the response I was given when I asked on ChatGPT, “What is your role as an AI in finance?” What I am hearing is that the advancement of Artificial Intelligence (AI) has scaled such exponential heights that it might seem daunting to many of us out there. But that is the reality of today.
What exactly is AI in finance, then? By definition, it is the use of technology like machine learning (ML), a branch of AI and computer science that focuses on the use of statistical models and algorithms to mimic human learning and gradually improve its accuracy, to enhance how financial institutions analyse, manage, invest, and protect money. In fact, most banks are highly aware of the potential benefits presented by AI. The decision for financial institutions to adopt AI will be accelerated by technological advancement, increased user acceptance, and shifting regulatory frameworks. For instance, by providing customers with 24-hour access to their accounts and financial advisory services, banks greatly enhance the customer experience and streamline laborious operations utilising AI.
A brief history of Artificial Intelligence
Let’s begin with the humble beginnings of AI in the financial industry. Though it might seem rather recent that AI has been a buzzword, it has always existed in some form or another, dating back many decades.
The Dartmouth Summer Research Project on Artificial Intelligence (DSRPAI), run by John McCarthy and Marvin Minsky, is where the phrase “artificial intelligence” was first coined in 1956. Despite widespread disagreement about accepted practices, the conference had a positive reaction overall to the idea of AI.
After World War II, there was a significant increase in paperwork, which increased the demand for systems to automate some of the jobs. Researchers did, however, predict the possibility of job losses brought on by automation, a perennial concern that continues even today.
Machine learning made extensive use of studies on Bayesian statistics in the 1960s. The popularity of Bayesian Theory is due to its use in the auditing field. The auditor bases their decisions during an audit on their professional experience. But diverse circumstances and unexpected occurrences necessitate different methods of decision-making. The Bayesian approach provides objective and logical probability to enable auditors to make assessments with greater accuracy. Also, it gets rid of errors in judgement brought on by the auditors’ own emotions. The cutting-edge technology was well received by professionals, and AI grew well into the 1970s.
In the 1980s, more than two-thirds of Fortune 1000 companies had at least one AI project under development. This was the era when AI began to gain prominence in the finance world. During this time, expert systems started gaining popularity as a great commercial financial solution. The financial sector utilised the Expert System, a knowledge-based intelligence system, up until the 1980s to forecast market movements and offer individualised financial strategies.
In order to lower the risks of human error, the banking sector and financial institutions started using expert systems more frequently. It supported corporate development, international trade, currency conversion, and bank management, in addition to financial and market analysis. In fact, in 1980, Edward Feigenbaum invested in expert systems that imitated a human’s capacity for judgement!
In summary, even before the 1990s, the development of AI in the financial sector was already very advanced. Dupont created 100 expert systems that enabled them to make annual savings of around $10 million. One of the earliest expert systems to predict the Dow Jones Industrial Average’s 87-point decline in 1986 was the Protrader system, which was created by KC Chen and Ting-peng Lian.
In the 1990s, the AI hype moved on to fraud detection. Around 200,000 transactions were evaluated each week by the FinCEN Artificial Intelligence System (FAIS). The algorithm might spot 400 possible money laundering instances totalling close to $1 billion over the course of two years.
Expert systems continued to be developed further right up to 2023, when they were built for the purpose of risk management to enable medium- and small-sized enterprises to conduct professional data security analysis due to capital constraints. Expert systems are no longer used in the banking industry. However, it has been crucial in launching AI and popularising its use in banking in the present era.
The role of AI in financial services today
The transition from expert systems to the form of AI we have today was a result of the limitations of the previous types of expert systems, urging researchers to develop new types of approaches. With the advent of machine learning and data mining approaches with feedback mechanisms, AI technology has become more efficient, flexible, and powerful at simulating the human decision-making process. Essentially, expert systems are applications that make knowledge-based inferences and decisions, while AI uses the concept of knowledge representation, which involves creating models and structures to represent information and knowledge in a way that intelligent systems can use, targeting to make machines intelligent.
In the financial services sector, the digital transformation of AI has been particularly apparent. In fact, banks and insurance businesses have been utilising AI for some time now and all players in Belgium, for instance, employ AI in some capacity, albeit to varying degrees. They employ AI in their business strategies and create roadmaps that change in response to technology, their goals, and their top concerns.
Process automation in back-office operations has led the way as well. The first tools, like the expert systems, allowed us to improve the ‘cost to service’ of operational functions. Initially, automated computational models that could make some predictions were the focus. But today, advanced AI that allows robots to generate rules automatically and acquire knowledge on their own is also available.
Combating fraud effectively
The use of AI tools in risk assessment has become more prominent. They help a bank foresee loan defaults or stop an insurance company from overpaying compensation. The battle against fraud and financial crime also greatly benefits from the use of AI tools. Banks operate within a highly rigorous regulatory environment, while fraudsters’ resources and creativity are also constantly developing. But thanks to AI models, we can now identify unusual activity and combat fraud more effectively.
The models that are used to further define “client risks” are not just based on financial ratios and the inherent traits of unusual transactions. Artificial Intelligence automatically analyses, aggregates, and integrates vast amounts of external public or purchased data. It includes data from the financial media as well as an expanding range of market data.
Algorithms to automate lending
Lending is one of the areas of finance where AI is most frequently used. Businesses can assess customers’ creditworthiness when they apply for credit cards and other loans by using machine learning algorithms and pattern recognition, which go beyond the standard review of credit scores and credit histories.
Platforms for AI lending, such as those offered by Upstart and C3.ai, can assist lenders in approving more borrowers, lowering default rates, and lowering the possibility of fraud.
According to C3 AI, its platform for smart lending lowers borrower risks and streamlines the loan origination process for financial institutions. For instance, it claims to cut the time needed to approve a loan applicant by 30%. Also, it increased the number of applications and loan acceptance yield by $100 million.
Shares of Upstart have crashed as its growth has slowed due to rising interest rates, the banking crisis, and increased pressure on borrowers. But there is no need to question the AI technology that powers this company, as both AI and machine-learning algorithms are built to draw conclusions and make decisions based on vast volumes of data.
Investing advice from AI
In order to provide customers with stock recommendations and content, investing platforms are also utilising AI.
Among financial stocks, Robinhood is probably the epitome of this type of platform. The well-known trading software has employed AI to set itself apart from rivals and suggest investing opportunities based on factors such as risk tolerance, investing style, and history. The user experience is made more personalised by AI features.
Magnify is a new app that advances AI further by integrating ChatGPT and other tools to provide individualised financial advice, much like ChatGPT can be used as a copilot for coding. Magnify also functions as a trading platform, allowing users to place trades and providing information on stock performance.
A personalised touch to customer communication
Financial services are gradually learning how to use AI in another way: by gathering data and improving the customer experience, they can better understand their customers and their behaviours. Technologies that analyse the text and audio of customer conversations enable the development of “chatbots”, which are now commonplace on most websites.
Will we eventually get proactive, tailored AI-generated recommendations for new savings, investments, or insurance products? ChatGPT and generative AI in general have enormous potential for financial services at many strategic and operational levels. With generative AI, you can communicate with customers in greater depth and with great added value without needing to have a lot of technical expertise. You can create a variety of answers using different media types, including text, images, sounds, and videos, in any language and with your own communication style.
Most importantly, the technology enables us to continuously automate several background processes even as customer interaction is taking place. Creating a contract, determining the warranty, or figuring out the appropriate business discount while gauging customer satisfaction through facial reading and voice listening The account manager’s job will be made simpler as a result. Although technically conceivable now, the financial services industry is adamant about keeping control.
The ‘augmented’ banking or insurance consultants will be the customers’ most noticeable prospects. They are helped by AI to interact with customers more quickly and in a more personalised style. Belfius is among the many other players in the market using this strategy.
Drawbacks of AI
While the perks of AI are far-reaching and profound, its use can also cause imbalances if uninhibitedly unleashed. Let’s start with the rise of robo-money managers: It is very possible for Artificial Intelligence to bring about major disruptions to the wealth management industry, already steeped in heated competitive battles for fees and inflows.
One in six asset and wealth management firms will be acquired or shut down in the next five years, according to a recent PwC poll of asset managers and institutional investors. On AI’s impending impact on the wealth management sector, pundits reckon that the “tech carnage” could be significant. If smaller shops do not look out for each other, they will be forced to consolidate and invest in a lot of game-changing technologies that will replace existing firms.
Where is robo-advice now? This game-changing technology is starting to be seen in sophisticated AI-powered models that educate investors on wealth-building actions today and retirement planning for the future. Acorns, Betterment, Fidelity (FIS), Schwab (SCHW), and Vanguard are among the few businesses that already provide and invest in robo-advice services.
The powerful will get even stronger, not only because they have the technological vision but also because they have the enormous resources to carry it out. According to PwC, these investments are accelerating the rise of robots. By 2027, the company projects that robo-advisors will have grown to oversee a staggering $6 trillion.
More investors are following this digital investment advice, and recent dealmaking shows that money management organisations are under pressure to expand in order to pool assets and technology and weather the storm. In fact, 341 Merger and Acquisition agreements were completed in the wealth management sector last year, according to investment bank and consulting firm Echelon Partners, which is an 11% increase from 2021 and the largest in at least ten years.
Wealth management is likely to change through a “barbell strategy”, where big players will continue to scale and smaller, more niche organisations will need to be innovative and idiosyncratic to succeed. At the end of the day, it will come down to who can adapt to the robots the best and avoid being devoured alive.
So regarding AI, yay or nay?
With the infinite possibilities that Artificial Intelligence offers the world, there is no doubt that it will be here to stay. In the past couple of decades, we have witnessed AI evolve at an exponential speed, from the “dated” expert systems, which at present might not even qualify to be labelled AI anymore, to commonplace applications like Siri and Alexa which are virtual assistants capable of performing recognising speech, processing it as data, and then responding with an answer or action. Something that was merely science fiction not too long ago.
As human-like as AI is today, it would still be solely up to us to decide to what extent AI can take over some of our roles, as well as how much more efficient it can be. Would it also be worth the cost? The fear of being entirely replaced might not be as necessary because, ultimately, AI solutions cannot always guarantee the level of precision required by compliance, nor can they replace human judgement grounded in sensitivity and experience. However, this shouldn’t discourage companies from using AI. It can definitely be used in conjunction with humans as a tool to empower professionals and facilitate the process of regulatory compliance.
Trader’s View
As of today, the AI that we are all familiar with, whether it be ChatGPT, Google Bard, or other generative AI software, is still in its infancy. Despite its extensive history, widespread use was not accessible throughout the 1960s and 1970s. Companies like Microsoft, Google, Nvidia, and many others have just recently been able to incorporate technology into everyone’s everyday lives due to technological innovation. It is not difficult to predict that in the near future, all of us will have an AI assisting us in our everyday lives in some way.
Numerous industries, including healthcare, banking, manufacturing, and entertainment, stand to benefit significantly from AI. It may improve user experiences, production process efficiency, financial trading strategy optimisation, and medical diagnostics. The impact on jobs and ethical and societal issues like prejudice in AI algorithms need to be carefully considered.
Continuous research and development are essential to unlocking AI’s full potential while guaranteeing a responsible and ethical application as it continues to grow.
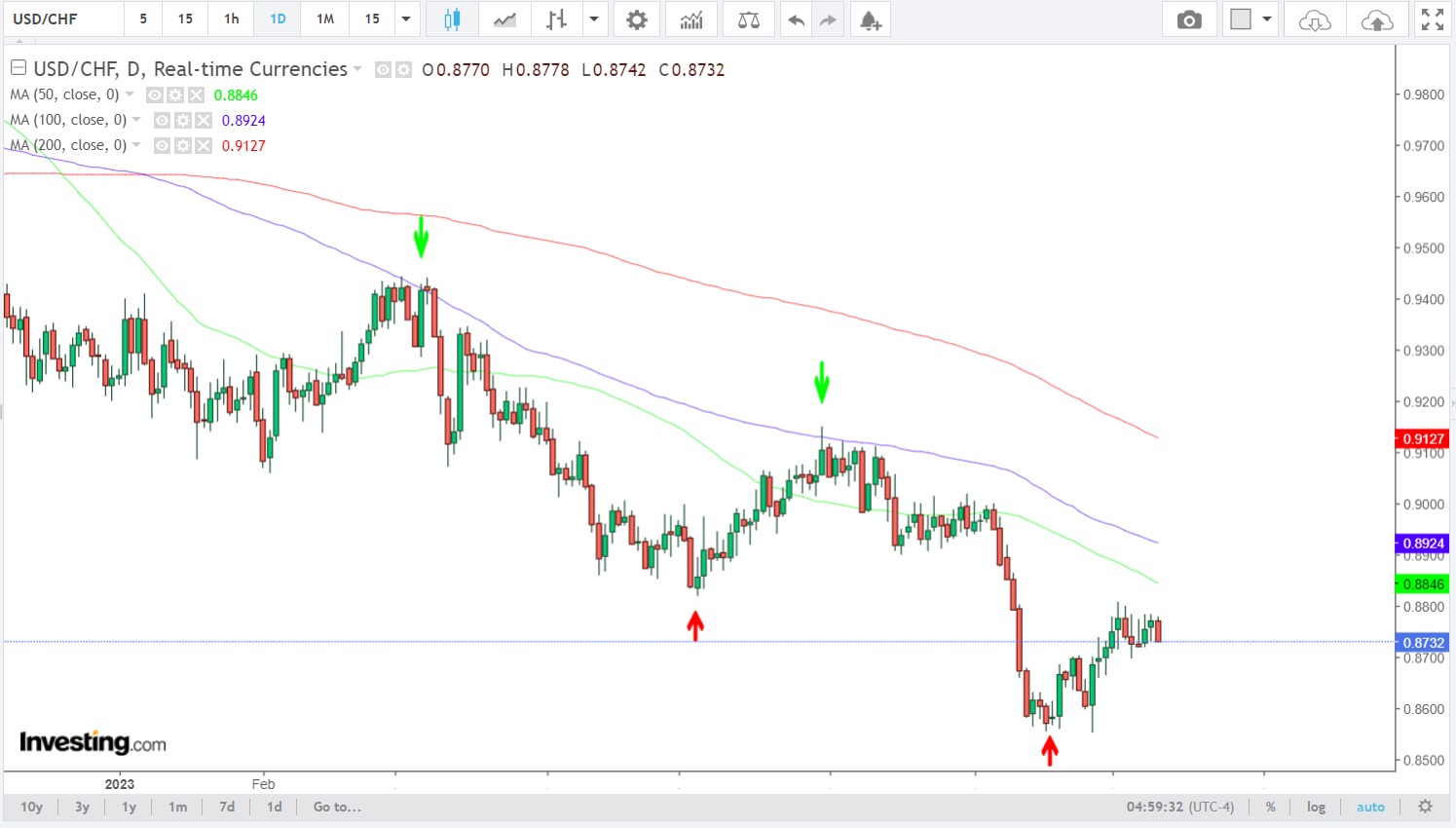
This month’s currency pair will be USD/CHF. Based on technical analysis of currency pairings such as USD/JPY, EUR/USD, GBP/USD, USD/CAD, and USD/CHF. This month, the USD/CHF offers an excellent setup for trading.
The chart above is a daily chart. At first glance, we can observe that the USD/CHF has been declining since early February 2023. The green arrows represent lower highs, while the red arrows represent lower lows. Following that are the moving averages in green (50 MA), purple (100 MA), and red (200 MA). The 50 is below the 100 MA, while the 100 MA is below the 200 MA. These three MAs are in the right sequence. Based on these two variables, we can confidently assume that the USD/CHF is in a downtrend.
At 0.8921, or the 100 MA level, is a suitable entrance level. Additionally, the two most recent lower highs retraced at 100 MA, confirming that 100 MA is an excellent resistance level. The next entry level would be at the 0.8847 level of the fibonacci retracement at 61.8 percent. Only take into account this price range if the price is hovering around 0.8800 and a bearish candlestick pattern has been confirmed. Our take-profit point will be somewhere near 0.8500.
Basil Goh